Advice for investors: what to bear in mind when looking at AI startups
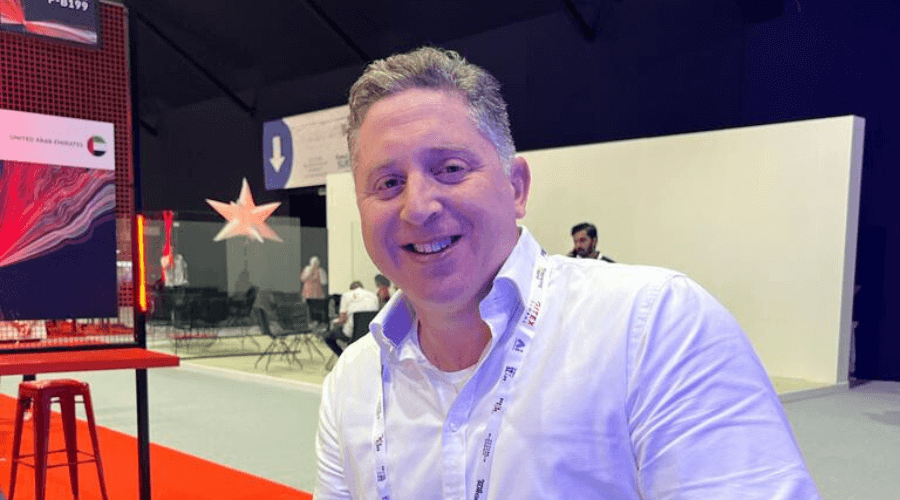
Ayman Alashkar, is the founder of UAE-based proptech startup OVERWRITE.ai
There are those who are calling AI a bubble. When AI-generated pizzas are touted on your local food delivery app, it's tempting to agree.
Many investors are struggling to see pathways to AI profitability through the noise. Here are a few simple rules of thumb any investor should apply to their AI startup selection strategy:
Naming rights
It is NOT all in the name. Artificial intelligence is the process by which we teach computers to behave and reason, as humans would. To understand problems and to solve them, by and for themselves. There are two types of artificial intelligence - narrow AI and general AI. It is important not to mistake generative AI with general AI.
General AI is where machines are able to outperform humans at all tasks. We have not developed any such technological capabilities yet, and the jury’s out on when or if we will anytime soon.
Narrow AI is where machines are able to learn and improve their performance of a singular task. Open AI’s ChatGPT is a conversational AI bot built on the GPT Large Language Model (LLM). Google’s Lamda is another such LLM. Both are examples of narrow AI, designed to generate content, whether generating text, images, or genomic sequencing, as well as humans. Or better.
Many companies are now calling themselves AI this or AI that. Others claim they have incorporated AI into their processes.
But you need to get under the hood of these companies. Understand what problem their AI is intended to solve and the process it is applied to.
Integrating a narrow AI system such as a Large Language Model into a process may sound smart, until it isn't.
Take the recent instance where UAE restaurant delivery service Talabat integrated ChatGPT into a customer-facing role, only for it to recommend Talabat’s competitor Careem to their users.
Because LLM development has mostly been the domain of the super-funded tech giants to date, companies that plug-in to them have little or no control over the source data used to train them.
And in the rush to embrace AI, even tech-enabled businesses like Talabat are tripping up. If they can get it wrong, others will too.
Scraping rights
Whoever thought they could trawl the internet for other people’s content, and repackage it as their (AI’s) own, was probably getting very shrewd, or very bad legal advice.
When a Large Language Model is trained and tested on a corpus of content created by others, then logic and copyright laws suggest the creators have rights to it. Some such people are making themselves known, and their grievances heard.
The comedian Sara Silverman who recently sued both Meta and OpenAI for breach of copyright, represents just the beginning of a wave of legal blowback from content creators who do not approve of their material having been used and resynthesised by Large Language Models.
It is becoming more essential for companies to build their proprietary LLM, train it on its proprietary corpus, to avoid the potential of a future where its input gets its output into legal hot water. Doing so allows one to become a truly asset-backed generative AI, completely independent of third party and copyright infringement risks.To the investors out there now getting in on the AI trade, copyright risk matters. A LOT!
Take the long road, not the shortcut
Short-term thinking is anathema to prudent investment. Many investors today are facing higher costs of capital and are therefore looking to revenue generating investments as a risk-mitigant solution. Sensible. Except in the context of the emergent AI opportunities on offer. In the AI context if you're looking for revenue today, you're being short-termist.
Remember that in 2015, OpenAI was funded with $1billion at Day 0, (with over $10billion more since). It is still not profitable.
Take the long road. Look to the cross-fertility of a startup's data. Understand what is proprietary about its tech stack. These assets can be collateralised, even if they are not yet revenue generating.
The metrics you should use to assess an AI investment opportunity, will be new to you. They are not going to be the same as you have seen before.
Risk on, risk off
Sound investment is not about maximising profit. It is first and foremost about mitigating risk. AI hallucination and model collapse risks are very real downsides to the future health of any AI startup.
Hallucination is when Large Language Models make things up in order to deliver output, whether accurate or not. In use cases where high accuracy is necessary, LLMs that are output-oriented will, over time, prove inferior to their domain-specific alternatives that prioritise outcomes over output.
Likewise, a Large Language Model trained on AI-generated content is at very real risk of qualitatively degrading its own output. Think of the blandness of future content that we rely upon, when we recycle AI-generated content in a model’s feedback training loop. The outcome will see models collapsing under the weight of exponentially homogenised input data.
Understand and quantify the risks that your AI carries. What can be done to mitigate them? Are you prepared to accept them?
Size does matter
LLMs require enormous amounts of capex to build, and opex to run. But even that does not mean that well-funded labs can continue to grow models in perpetuity.
There comes a tipping point when, even with hardware advancement, there simply is not enough power to cope with ever increasing model size. As a result, the only way most people can access the most powerful models is through the APIs of tech giants.
That creates a dependency risk; exposing businesses to the decisions that others take on how to build, train and power their models. There is a quietly growing trend towards smaller models that are purpose-built and domain-specific, and therefore cost less.
By creating LLMs that are themed into a specific sector, it is possible to deliver high accuracy output and all the value therein, without the enormous costs associated with it. They present a lower risk opportunity to invest in AI. It is important not just in eliminating dependency risk, but to ensure the business is asset-backed by a proprietary tech stack.
What the Mena startup ecosystem needs
Investors that look for traction from a startup in an industry that is, in itself, still finding its feet, are making a mistake.
The fog surrounding the AI investment landscape will not be cleared with conventional touchstones. Bland metrics like traction are not reliable markers for Mena’s investor community of an AI startup’s potential, because they do not paint the complete picture.
Instead, investors need to look for people who have been in the AI trenches to help them define risk and mitigate for it. People who have created AI systems, rather than merely plugged into and fine-tuned them. Experienced hands, who know what AI hallucination looks like without having to wait for it to happen, who know which processes require which AI applications, and which do not.
To separate the truth from the snAIk oil, it is not enough to just know the difference between general AI and generative AI.